In a previous article, I talked about how shooting variance can have a huge impact on single games. This is still very true, and we can see the effects of it on a night-to-night basis in NBA and college basketball games. However, in the article I also mentioned for a sentence or two that shooting variance can have a massive impact on entire seasons. In this article, I am going to be diving deeper into that statement, and hopefully provide you with some visual evidence to help you further understand how much of an impact shooting variance can have.
The inspiration to write an article on this is stemmed from nerd Twitter. A frequent discussion is about the New York Knicks and their success so far this season. Per Cleaning the Glass, they have the third best defense in the NBA. However, some of this can be chalked up to shooting variance. The Knicks rank 29th in opponent location eFG%, which is how well the other team would shoot if they shot average from each area of the floor. They give up a lot of shots around the rim and three's, but don't give up a lot of long twos. While shooting percentage at the rim is something a team can impact because of scheme or personnel (the Knicks have two good rim protectors in Noel and Robinson), opponent shooting percentage from 3 is usual more of a luck-based metric. Knicks opponents this season are shooting a league worst 33.2% from 3. If you're not convinced the Knicks aren't just getting the benefit of shooting variance, then take a look at this stat: the Knicks are giving up the 5th most open threes per game at 18.8, but their opponents are shooting by far a league worst 33.4% on those attempts.
Side note: the Knicks have the 24th ranked offense and the 3rd ranked defense. Is Julius Randle driving a team to the 24th ranked offense enough to make him an All-Star? That team wins with defense, and Randle is not the one winning them games. Give me a break...
In the NBA, we work with small samples. 82 games may seem like enough, but it is not a large enough sample to give us an accurate standing that depict how good the teams are. It may allow for the very best and worst teams to stand out, but the one's in the middle can greatly shift around because of shooting variance. To show you this in a nice visual way, I used some programming and math to simulate a season. I took how many times on average a team shoots from a given range, the average percent they shoot from that range, and had them "play" against an opponent with the exact same profile. The program had the two teams go against each other, with each team shooting the same number of shots, from the same locations, with the same likelihood of the shots going in. I first did this for 30 teams, each playing 82 games, and here were the results:
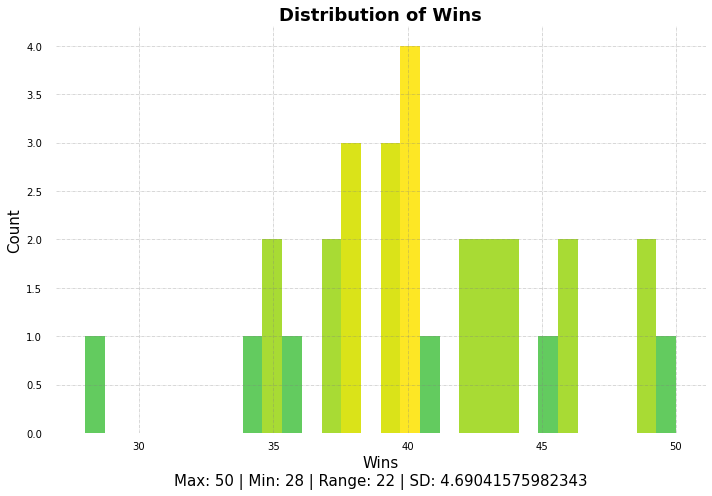
Pretty crazy right? Over the course of an 82-game season, with each team literally being the exact same, there was a huge degree of difference between the records of some of these teams. Since I made all the teams average, we can say that we should expect any one of these given teams to win half of their 82 games, which is 41. Only 1 of the 30 teams ended up winning 41 games, but 4 teams did win 40. The crazy part is that one team won 50 games while another team won 28. I simulated one single season, with every team shooting the exact same shots for every game, and the results showed a lot of variance. If 82 games aren’t enough to judge a team, definitely don't judge one after 30.
To show the effects of this a little but more, I wanted to simulate 1,000 seasons like this:
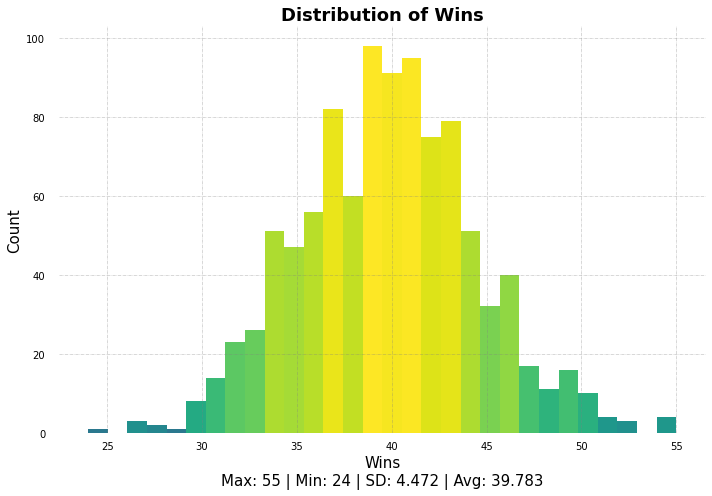
Did I want to see how low a team could go? Yes, of course. Some teams finished with only 24 wins, 17 less than expected. Another few teams finished with 55 wins, 14 more than expected. While the expectations are a bell-curve, that doesn't mean that there isn't a whole lot of variation of different outcomes. The range here is 31 wins, which is a crazy number for only 1 82-game season.
Of course, I wanted to do another one of these, but this time with 10,000 seasons simulated so we can actually use the numbers:
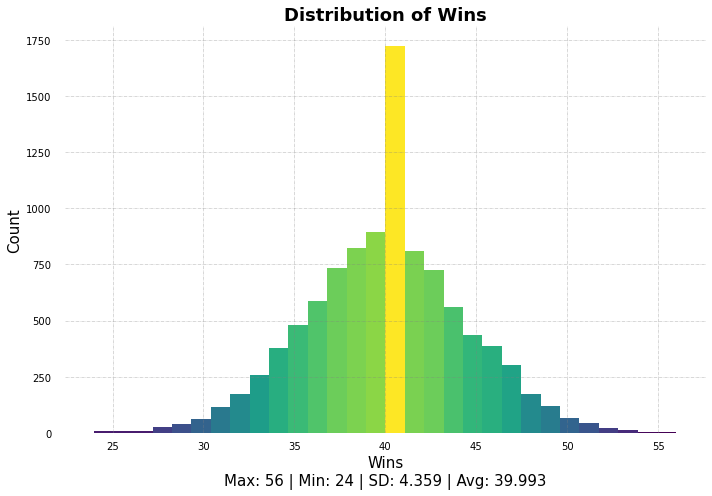
No team decided to dip below the 24 number, but a team did get all the way to 56 wins. With this graph, we have a beautiful standard deviation number that we can use to find percentile outcomes. With these simulations, a 50th percentile outcome would be about a 40-win team (just the average). When busting out my good old friend the Z Score table, we find that a 75th percentile outcome is about 42.766 wins, and a 25th percentile outcome is about 37.219 wins. Over the course of the season, a difference of about 4.5 wins depending on how shooting variance treats you is absolutely massive. For reference, in the 2018-2019 season (the last season with 82 games), the difference between the 3rd and 9th seed in the West was only 3 games.
Obviously, since I made this tool, I wanted to play around with it a little. For all the idiots that say analytics tells teams to chuck three's, you're wrong. This is what would happen if an NBA team only shot at the rim and from the free throw line over 1,000 seasons:
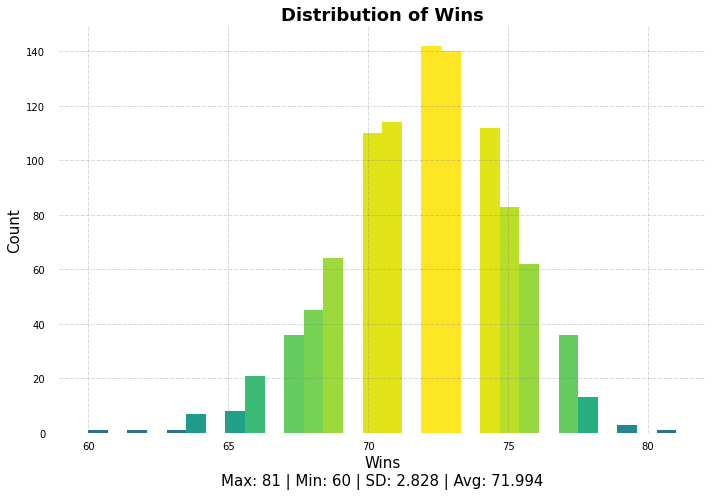
Yeah, they would average about 72 wins. One team won an insane 81 games out of 82. The worst team only won 60 games, which is still pretty good. If teams could get to the rim or free throw line every single play, they would. It just so happens that the three-pointer is the next best option after that.
For something a little more realistic, this is what would happen if we redistributed all of our mid-range shots into only shots at the rim, from the free throw line, and from three over 1,000 seasons:
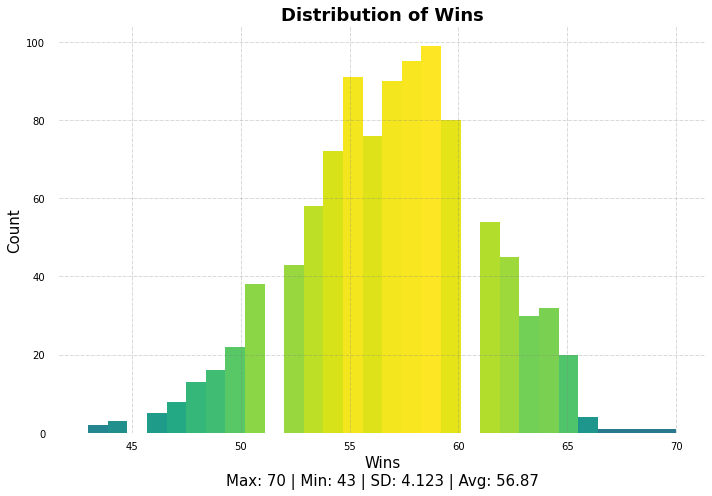
Still pretty good. Maybe not as good as only shooting at the rim or the line, but an average of almost 57 wins is a proposition I'm sure teams would be happy to take.
Obviously, this would never happen, but what if we only took only long mid-range jump shots over 1,000 seasons:
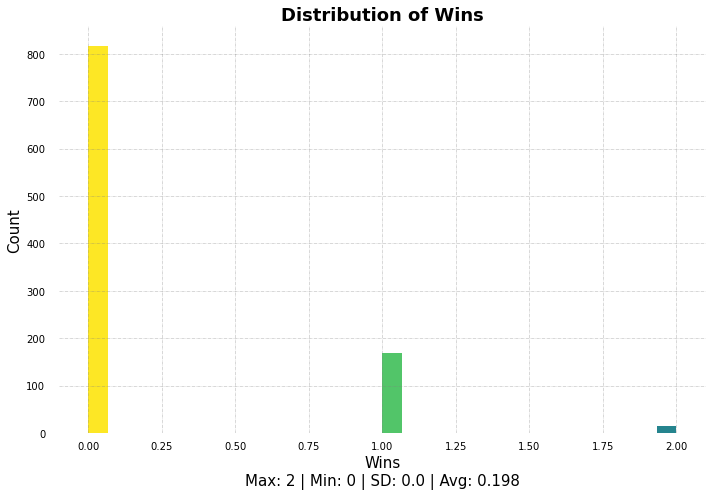
Yep. Over 80% of the teams won zero games over the 82-game season. A few teams did get all the way to two wins though.
Now onto something that is actually completely realistic. What if we took half as many shots from long mid-range, and instead evenly redistributed those shots with looks at the rim and from above the break:
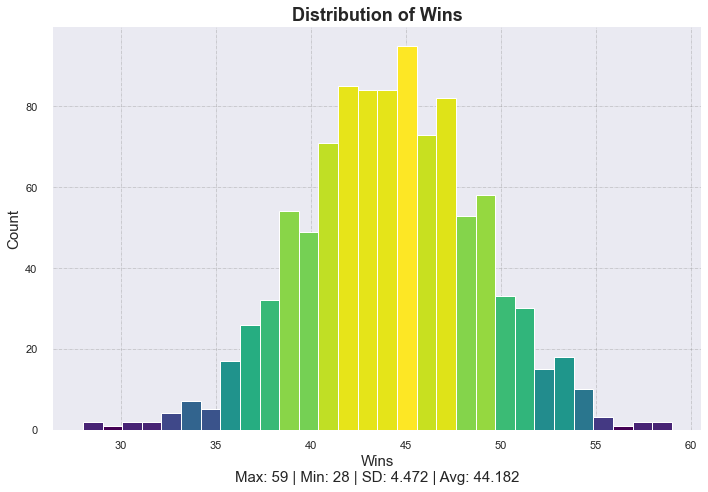
Using a fairly realistic strategy, we increased our average wins by about 4 games. Not only that, but the standard deviation is the exact same, meaning we wouldn't necessarily expect more variance in the outcomes if we did this strategy. And this is something super realistic that teams have been doing for years. Right now, teams shoot 11% of their shots from long two, and I would expect that to continue to go down. The value proposition is just too great.
But wait. The same gains and losses can be applied in the exact same way to our defense too. I already talked about how important at the rim looks can be for your own offense, but they are also vitally important to the other team’s offense. The graph below looks at the outcomes if we did the same offensive strategy as above, but defensively allowed 5 less shots at the rim and 5 more shots from above the break:
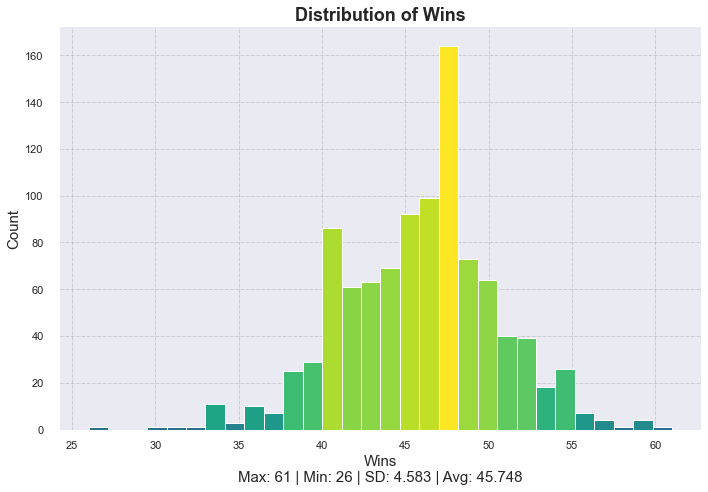
Here, we gained about 1.6 wins. Which is absolutely a massive difference. Some teams have already been smart enough to make this trade off, with some examples being the Bucks, Raptors, and Heat. Strategically, this looks like teams either executing a drop coverage with a good big man like the Bucks or teams collapsing on drivers and bringing harder help from one pass away like the Raptors. Gains like these are exactly they types of things that can help any basketball team win at any level. Just take a look at what Nate Oats has done in Alabama.
I also want to briefly talk about how this applies to playoff basketball. There is a lot of people who will sit here and say that the winner of a seven-game series is the better team almost 100% of the time. This just isn't the case at all. The graph below shows the outcome of the first 4 games of the series of two exactly equal teams if played 1,000 times:
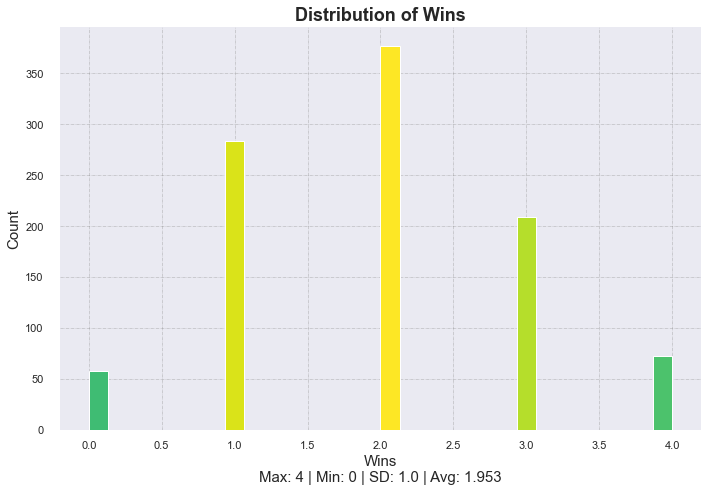
The series after 4 games is going to end up tied less than 50% of the time. in about 12.5% of the series, it's already over after 4 games despite the two teams being exactly equal. We can get to these same numbers with a simple thought experiment. If two teams play each other that are exactly equal one time, then one team will win. The odds of either team winning are 50%. The odds of a team going up 2-0 in the series is 25%. The odds of a team going up 3-0 is 12.5%, and at that point the series is essentially over. The odds of the other team coming back would be 6.25%. We can do this same thought experiment for a series tied 2-2. If two even teams are playing a seven-game series and it's tied 2-2, there is a 50% chance of either team ending up leading 3-2. At that point, they would have a 75% chance to win the series, while their opponent only has a 25% chance to win. Just because of basic probability, the best team does not win 100% of the time. Not even close. If 82 games aren’t enough to see who is actually the better team, then why would 7 be enough?
This is going to be my last point: shooting variance can have an impact on the way we see players entire careers.
The chart below shows the 3-point % of a player who played 15 seasons in the league, shot 350 three's a year, and every shot he took had a 38% chance to go in:
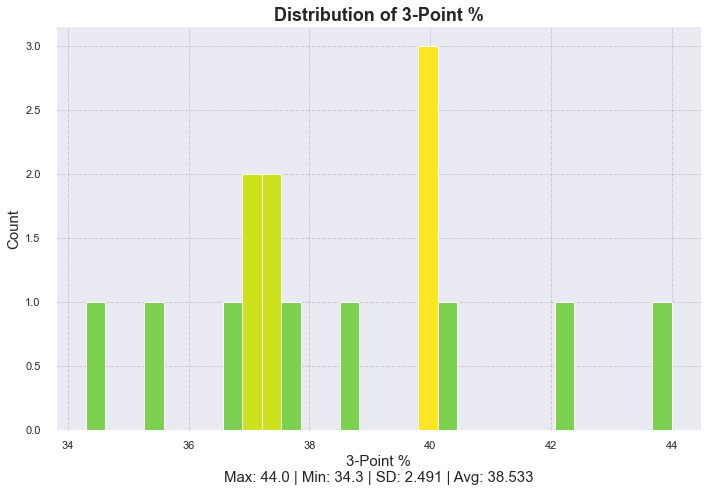
Pretty interesting, right? One year our player only shot 34.3%, but another year he shot 44%. And this is over the sample of 350 shots per season, which is a relatively high amount. Also, look at our players shooting percentage. We know he is actually a 38% shooter because I literally programmed him to be that way, but by history he will be remembered as a 38.5% three-point shooter, which is significantly better. Just remember this when you look at a player and think he got significantly better at shooting even though his offseason was only two months long.
Just for fun, what if our player was some kind of alien who played for 1 million seasons shooting the exact same number of times:

Our alien got all the way up to 52% one season and all the way down to 24.6% another. It only took him 350 million shots, but he ended up with a career 3-point % that reflected his actual shooting ability.
The code for this project can be found below:
Comments